The last few months we've spoken to more than 50 developers and data engineers, who work on Large language models (LLM)-powered apps. The shortlist of similarities we found about them is the following: they work on discovering LLM-powered applications, play a lot with prompt changes and model testing, few have active users yet, except for those who have build products for internal use.
If you are at a similar stage, below I would like to share 4 examples how LLM analytics can help you.
LLM analytics for AI app development
1. User request and AI reply logs
Above 80% of the developers and data engineers we spoke with did not have any type of analytics tool for their AI app yet. They knew that just keeping track of the LLM Q&A tests they are running would be helpful, but simply did not have time to work on such functionality.
Here are two examples how collecting request data can help you:
- When users start testing your app. collected data will help you see what they are asking and how the AI performs.
- In addition, on top of collected data of question and answers, you will be able to run other AI models, such as sentiment analysis, and see if users are happy or frustrated with the interaction of the AI bot?
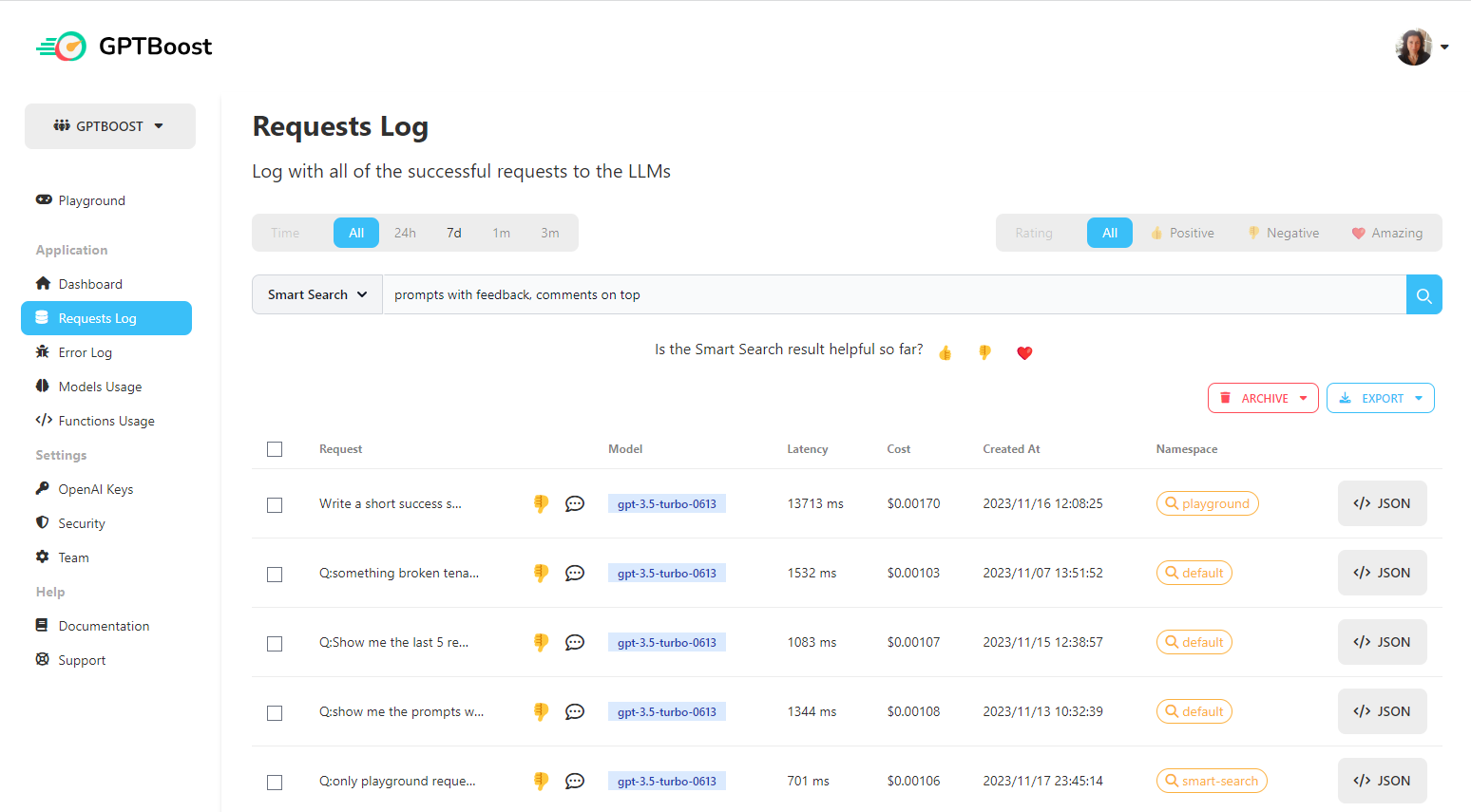
2. Prompt changes
Getting the prompt right is the toughest part in LLM product development. Developers and data engineers make 20 to 50 changes in the prompts on average weekly. The unpleasant part is that some changes prove beneficial for one aspect of the LLM output, but fail in another. That is why it is very important to keep track of the prompt changes you make and the progress they lead to.
Recommendations for prompt change process:
There are many articles and online courses discussing best practices for prompt engineering. Here is our recommendation how to track the efficiency of the prompt change:
- Identify 25 or so questions, which cover different use cases for the project your work on
- Test each new prompt with all 25 questions, so you verify the overall performance of the new prompt.
3. Model comparison
Developers test different LLM models to see which one performs best for their particular use case. But if they don't have clear comparison metrics, the results are uncertain.
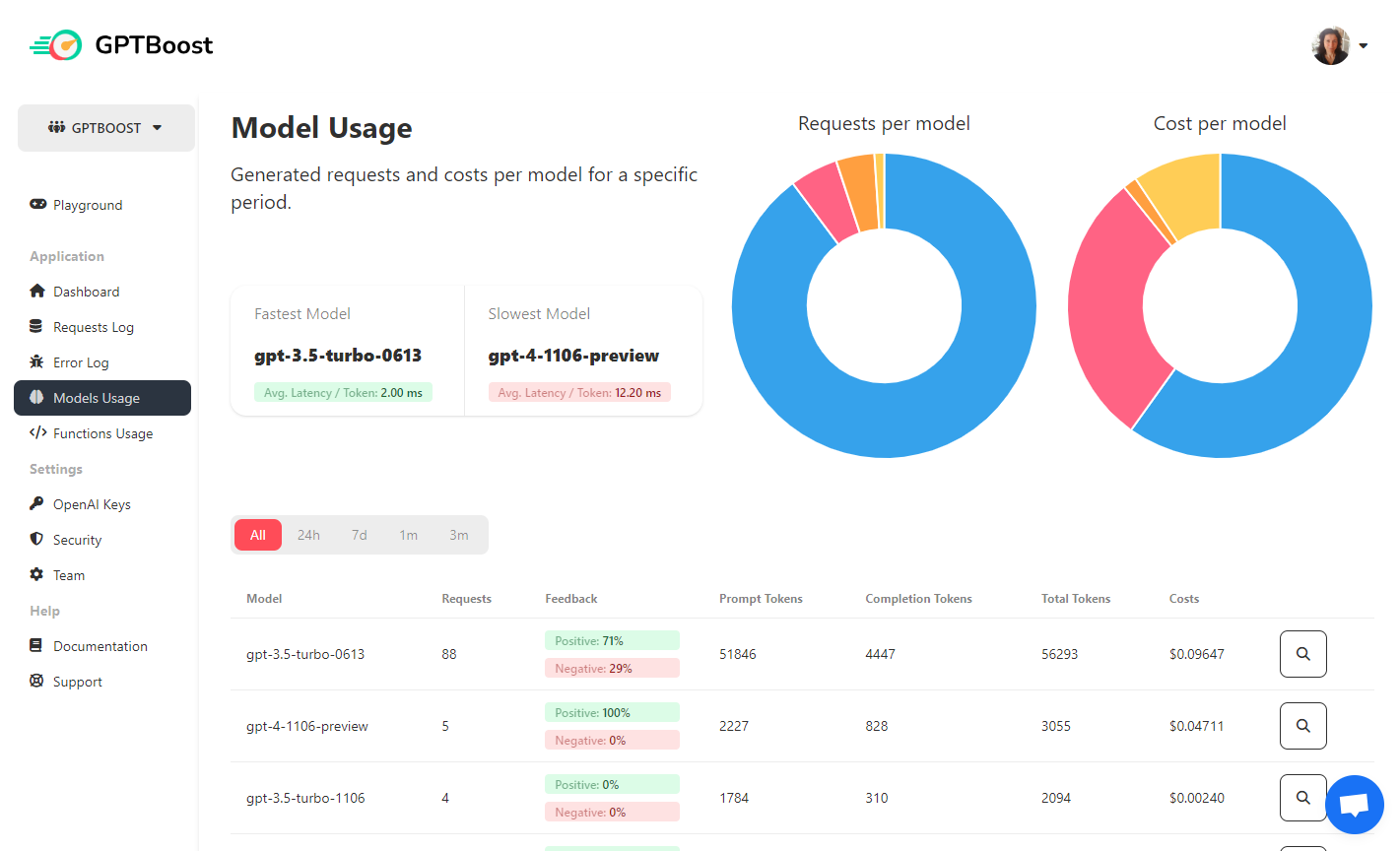
If you test different LLM models, the statistical tool can help you show price and number of requests run per model. Being able to compare LLM models is a great insight both for user experience and business decisions.
4. Error logs
Each prompt, which was not executed correctly by the LLM API, comes back with an error message. A quick review of the errors can help you debug your system quickly and continue with your project.
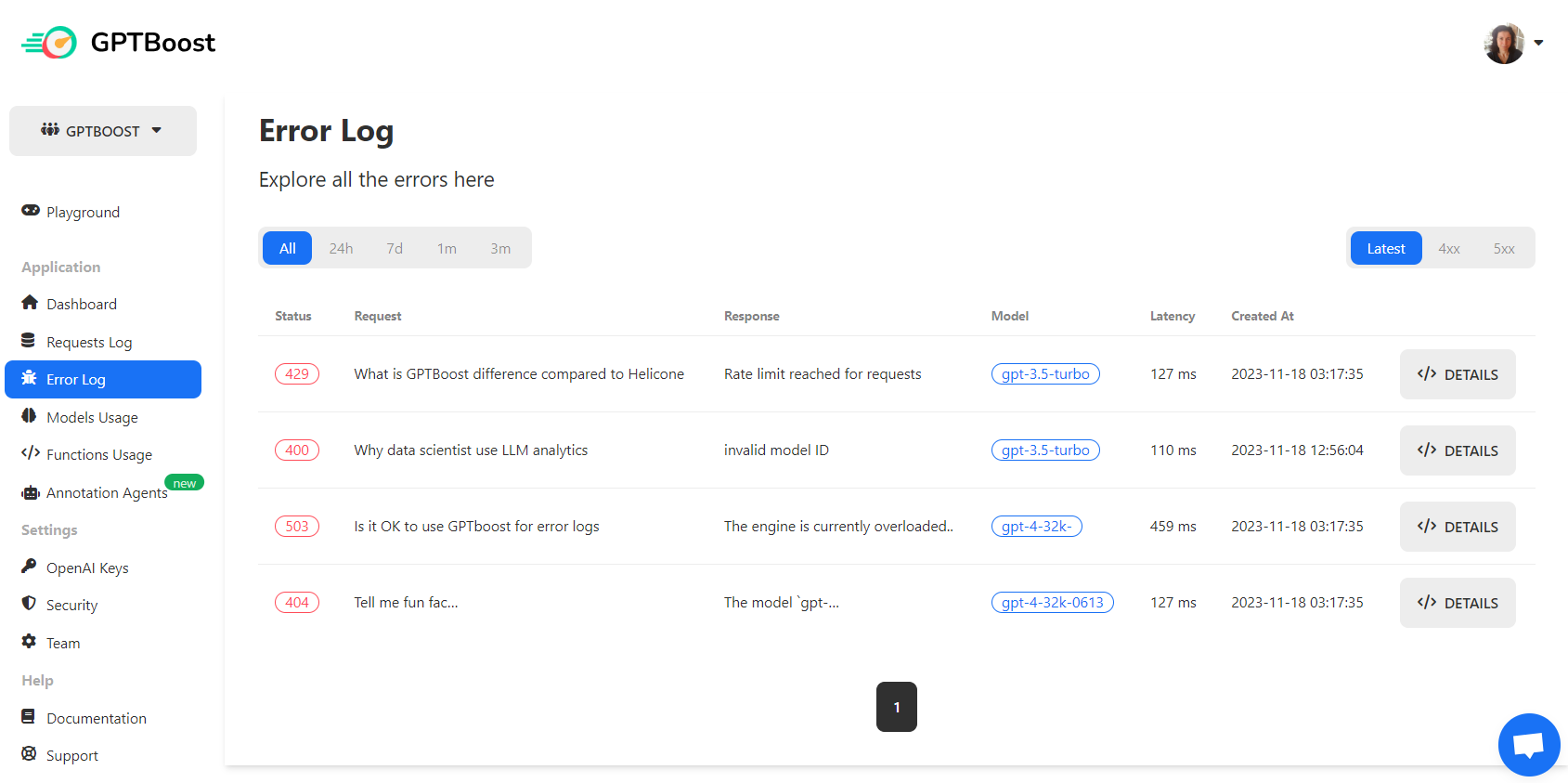
Analytics for LLM will help your AI app development and save you time and frustration, while revealing the insights that will help you improve your product and eventually lead to product-market fit (PMF). GPTBoost LLM analytics account is free to get started with: